Keynote Speakers
31st IEEE International Conference on High Performance Computing, Data, & Analytics
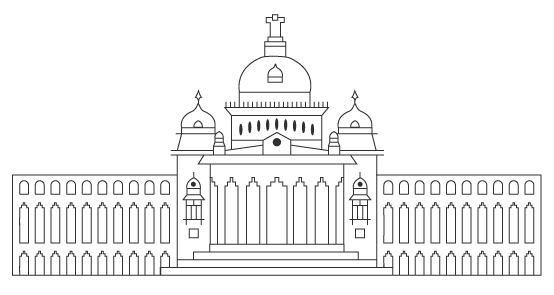
Keynote Talks
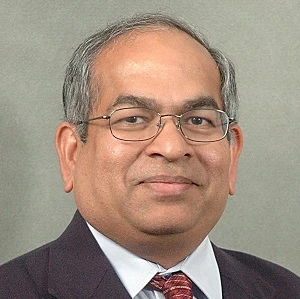
Title: Designing High-Performance and Scalable Middleware for the Modern HPC and AI Era
Speaker: Dhabaleswar K. (DK) Panda, Distinguished Professor of Engineering, Computer Science and Engineering, The Ohio State University, USA
Time: 19th December, 2024, 9 AM to 10 AM IST
Abstract: This talk focuses on challenges and opportunities in designing middleware for HPC and AI (Deep/Machine Learning) workloads on modern high-end computing systems. The talk initially presents the challenges in designing HPC middleware by considering support for dense multi-core CPUs, high-performance interconnects, GPUs, and emerging DPUs. Advanced designs and solutions (such as RDMA, in-network computing, GPUDirect RDMA, on-the-fly compression) to exploit novel features of these emerging technologies and their benefits in the context of MVAPICH libraries (http://mvapich.cse.ohio-state.edu) are presented. Next, the talk focuses on MPI-driven solutions for the AI (Deep/Machine Learning) domains to extract performance and scalability for popular Deep Learning frameworks, large out-of-core models, GPUs, and DPUs. MPI-driven solutions to accelerate data science applications like Dask are also highlighted. The talk concludes with an overview of the activities in the NSF-AI Institute ICICLE (https://icicle.osu.edu/) to address challenges in designing future high-performance edge-to-HPC/cloud middleware for AI-driven data-intensive applications over the computing continuum.
Speaker Bio: DK Panda is a Professor and University Distinguished Scholar of Computer Science and Engineering at the Ohio State University. He is serving as the Director of the ICICLE NSF-AI Institute (https://icicle.ai). He has published over 500 papers. The MVAPICH MPI libraries, designed and developed by his research group (http://mvapich.cse.ohio-state.edu), are currently being used by more than 3,400 organizations worldwide (in 92 countries). More than 1.8 million downloads of this software have taken place from the project’s site. This software is empowering many clusters in the TOP500 list. High-performance and scalable solutions for Deep Learning frameworks and Machine Learning applications from his group are available fromhttps://hidl.cse.ohio-state.edu. Similarly, scalable, and high-performance solutions for Big Data and Data science frameworks are available from https://hibd.cse.ohio-state.edu. Prof. Panda is an IEEE Fellow. He is a recipient of the 2022 IEEE Charles Babbage Award and the 2024 IEEE TCPP Outstanding Service and Contributions Award. More details about Prof. Panda are available at http://www.cse.ohio-state.edu/~panda.
Title: Utilizing LLMs for Parallel Scientific Code Generation and Translation
Speaker: Valerie E. Taylor, Division Director/ Argonne Distinguished Fellow, Mathematics and Computer Science Division, Argonne National Laboratory, USA
Time: 20th December, 2024, 9 AM to 10 AM IST
Abstract: Large language models (LLMs) have the potential to serve as an assistant to aid scientists with scientific discovery. One important area needed for this assistance is that of parallel, scientific code generation. Existing Large language models, such as GPT-x, Codestral, StarCoder, and Code Llama, etc. are being used to generate parallel, scientific code with some limitations. This talk will discuss some of the limitations and present our framework, called LASSI, to address some of the limitations. LASSI, is an LLM-based Automated Self-correcting pipeline for generating parallel ScIentific codes. Currently, LASSI features a pipeline that automatically generates code, tests for compilation, and checks execution. Future work will focus on code performance and correctness.
Speaker Bio: Valerie Taylor is the Director of the Mathematics and Computer Science Division and a Distinguished Fellow at Argonne National Laboratory. Her research is in high-performance computing, with a focus on performance analysis, modeling and tuning of parallel, scientific applications using AI. Her current work is on energy efficient methods.
Prior to joining Argonne, she was the Senior Associate Dean of Academic Affairs in the College of Engineering and a Regents Professor and the Royce E. Wisenbaker Professor in the Department of Computer Science and Engineering at Texas A&M University. In 2003, she joined Texas A&M University as the Department Head of CSE, where she remained in that position until 2011. Prior to joining Texas A&M, Valerie Taylor was a member of the faculty in the EECS Department at Northwestern University for eleven years. She is also the CEO and President of the Center for Minorities and People with Disabilities in IT (CMD-IT), for which she is a co-founder.
Valerie Taylor is an IEEE Fellow, ACM Fellow, and AAAS Fellow. Valerie E. Taylor earned her B.S. in ECE and M.S. in Computer Engineering from Purdue University in 1985 and 1986, respectively, and a Ph.D. in EECS from the University of California, Berkeley in 1991.
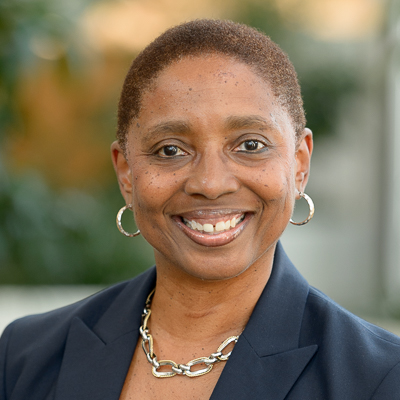
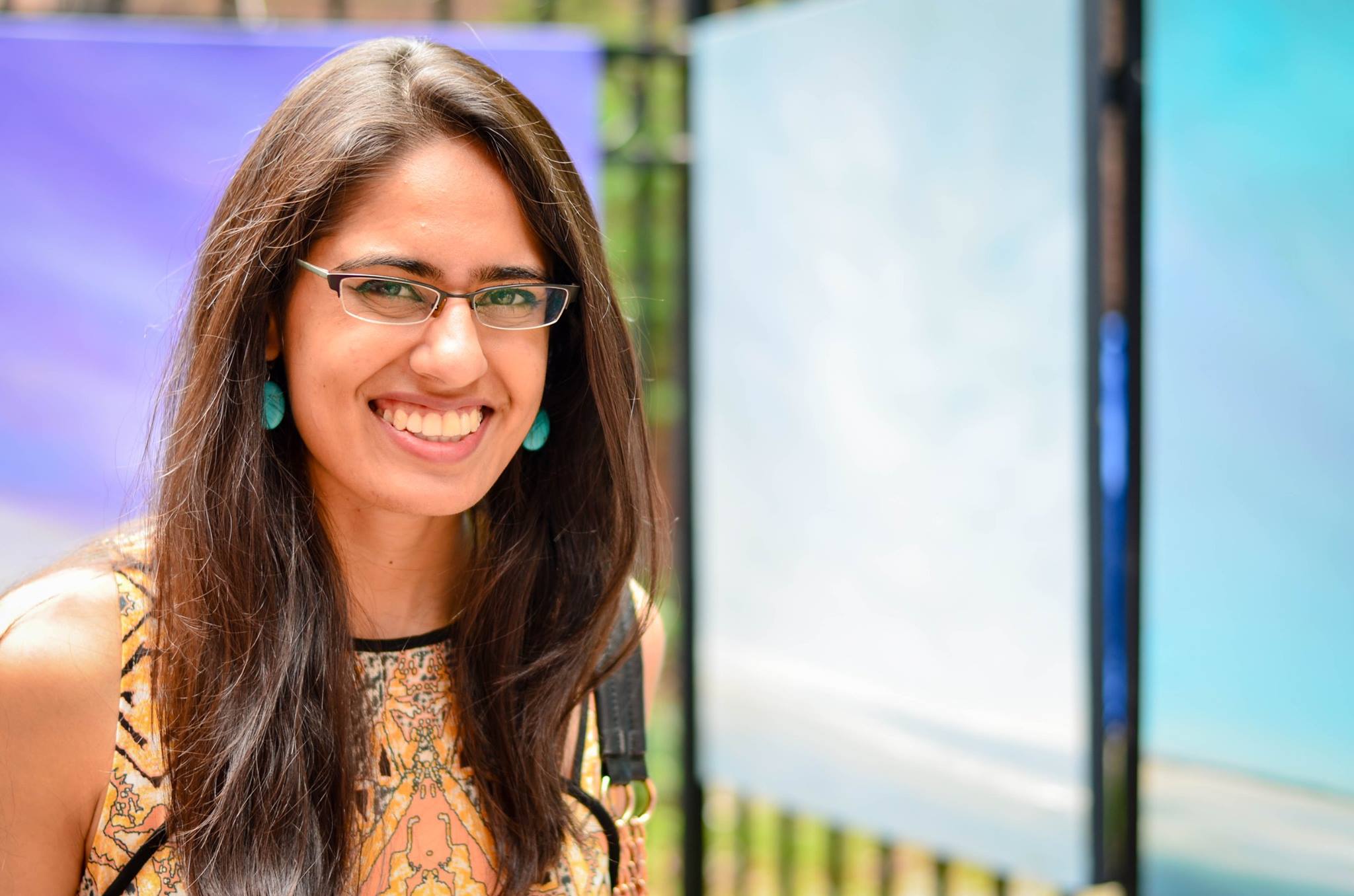
Title: Agile Design of Domain-Specific Hardware Accelerators and Compilers
Speaker: Priyanka Raina, Assistant Professor, Electrical Engineering, Stanford University, USA
Time: 21st December, 2024, 9 AM to 10 AM IST
Abstract: With the slowing of Moore’s law, computer architects have turned to domain-specific hardware accelerators to improve the performance and efficiency of computing systems. However, programming these systems entails significant modifications to the software stack to properly leverage the specialized hardware. Moreover, the accelerators become obsolete quickly as the applications evolve. What is needed is a structured approach for generating programmable accelerators and for updating the software compiler as the accelerator architecture evolves with the applications. In this talk, I will describe a new agile methodology for co-designing programmable hardware accelerators and compilers. Our methodology employs a combination of new programming languages and formal methods to automatically generate the accelerator hardware and its compiler from a single specification. This enables faster evolution and optimization of accelerators, because of the availability of a working compiler. I will showcase this methodology using Amber, a coarse-grained programmable accelerator for imaging and machine learning (ML) we designed and fabricated using our flow in TSMC 16 nm technology. I will show how we agilely evolved Amber into Onyx, our next generation accelerator, using an application-driven design space exploration framework called APEX enabled by our hardware-compiler co-design flow.
Speaker Bio: Priyanka Raina received the B.Tech. degree in Electrical Engineering from IIT Delhi in 2011, and the M.S. and Ph.D. degrees in Electrical Engineering and Computer Science from MIT in 2013 and 2018, respectively. She was a Visiting Research Scientist with NVIDIA Corporation in 2018. Since 2018 she is an Assistant Professor of Electrical Engineering at Stanford University, where she works on domain-specific hardware architectures and agile hardware–software codesign methodology.
Dr. Raina is a 2018 Terman Faculty Fellow. She was a co-recipient of the Best Demo Paper Award at VLSI 2022, the Best Student Paper Award at VLSI 2021, the IEEE Journal of Solid-State Circuits (JSSC) Best Paper Award in 2020, the Best Paper Award at MICRO 2019, and the Best Young Scientist Paper Award at ESSCIRC 2016. She has won the DARPA Young Faculty Award in 2024, Sloan Research Fellowship in 2024, the National Science Foundation (NSF) CAREER Award in 2023, the Intel Rising Star Faculty Award in 2021, and the Hellman Faculty Scholar Award in 2019. She was the Program Chair of the IEEE Hot Chips in 2020. She serves as an Associate Editor for the IEEE Journal of Solid-State Circuits and IEEE Solid-State Circuits Letters.
HiPC 2025 is the 32nd edition of the IEEE International Conference on High Performance Computing, Data, and Analytics. It will be an in-person event in Hyderabad, India, from December 17 to December 20, 2025.
IEEE Conduct and Safety Statement
IEEE believes that science, technology, and engineering are fundamental human activities, for which openness, international collaboration, and the free flow of talent and ideas are essential. Its meetings, conferences, and other events seek to enable engaging, thought provoking conversations that support IEEE’s core mission of advancing technology for humanity. Accordingly, IEEE is committed to providing a safe, productive, and welcoming environment to all participants, including staff and vendors, at IEEE-related events.
IEEE has no tolerance for discrimination, harassment, or bullying in any form at IEEE-related events. All participants have the right to pursue shared interests without harassment or discrimination in an environment that supports diversity and inclusion.
Participants are expected to adhere to these principles and respect the rights of others. IEEE seeks to provide a secure environment at its events. Participants should report any behavior inconsistent with the principles outlined here, to on site staff, security or venue personnel, or to [email protected].
Diversity and Inclusion
HiPC is committed to the promotion of diversity and inclusion in all professional activities. We encourage the diversity and welcome everyone regardless of age, gender identity, race, ethnicity, socioeconomic background, country of origin, religion, sexual orientation, physical ability, political views, education, and work experience.
Follow us on: